
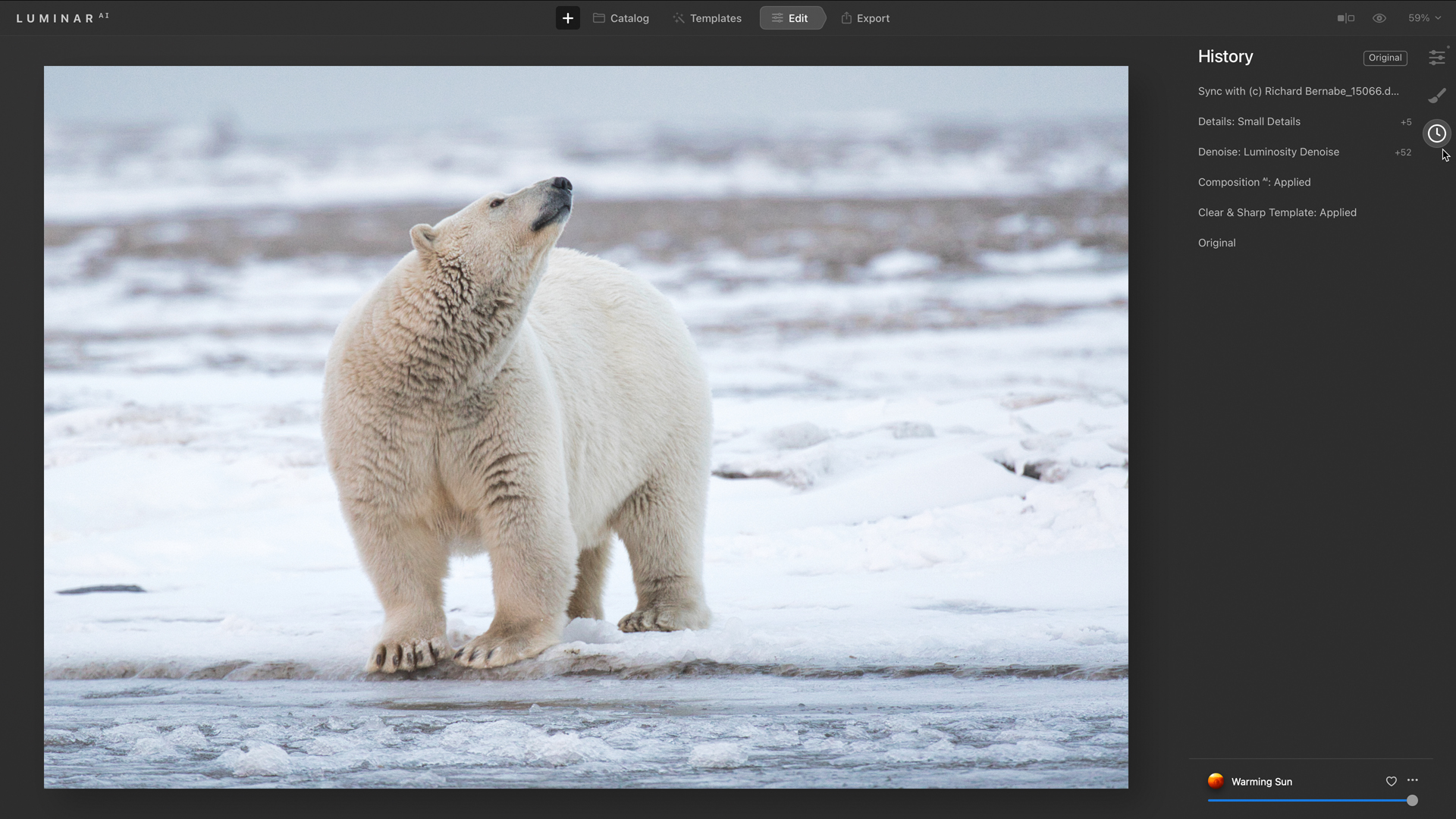
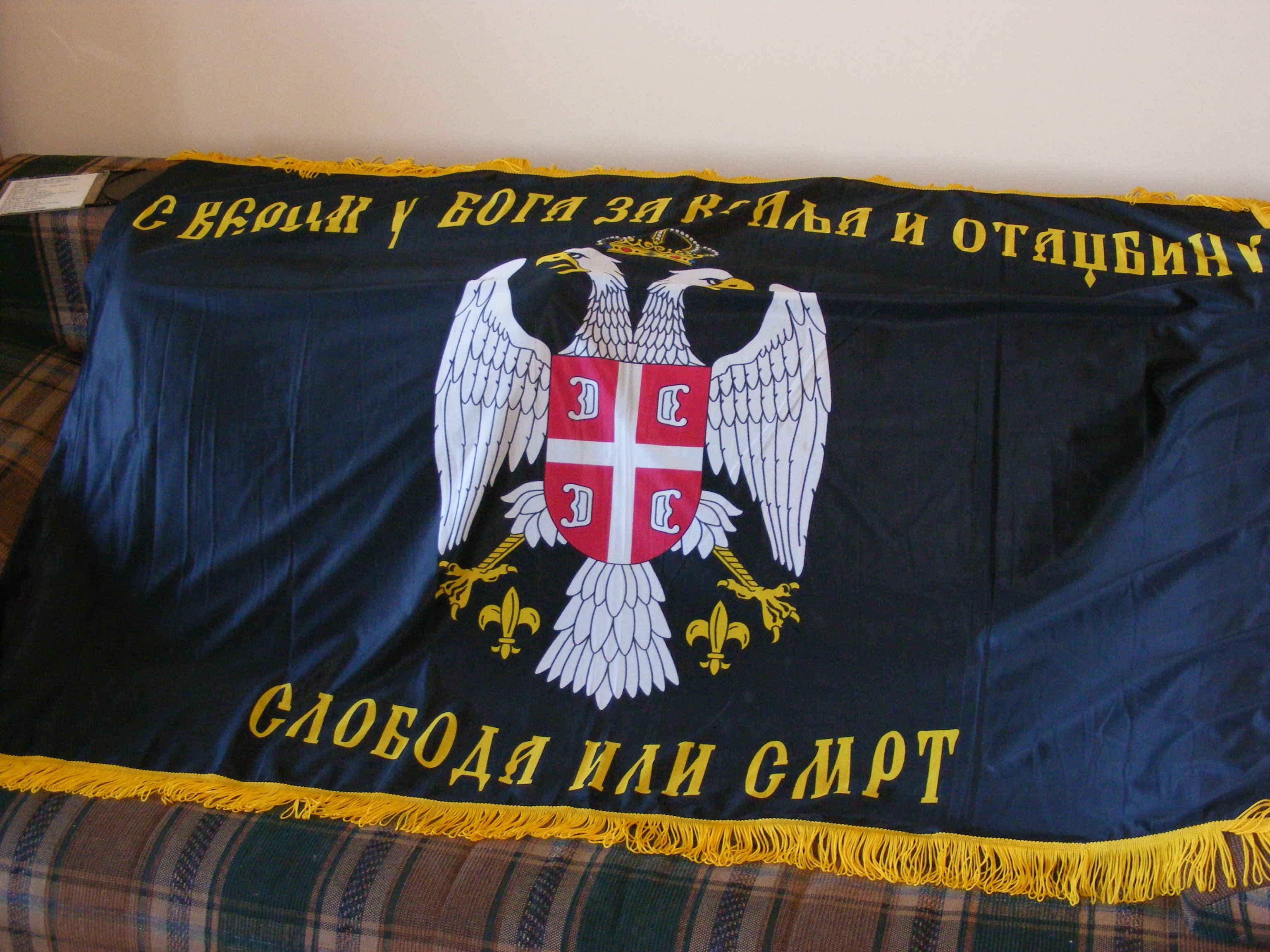
We demonstrate that adding mBART initialization produces performance gains in all but the highest-resource settings, including up to 12 BLEU points for low resource MT and over 5 BLEU points for many document-level and unsupervised models. Pre-training a complete model allows it to be directly fine-tuned for supervised (both sentence-level and document-level) and unsupervised machine translation, with no task- specific modifications. mBART is the first method for pre-training a complete sequence-to-sequence model by denoising full texts in multiple languages, whereas previous approaches have focused only on the encoder, decoder, or reconstructing parts of the text. We present mBART-a sequence-to-sequence denoising auto-encoder pre-trained on large-scale monolingual corpora in many languages using the BART objective (Lewis et al., 2019). Abstract This paper demonstrates that multilingual denoising pre-training produces significant performance gains across a wide variety of machine translation (MT) tasks.
